The tamping of switches and railway lines with tmA2, our track machine assistant has never been easier. With the recent upgrades we’ve boosted performance and enhanced reliability. We are excited to share all the details with you.
Support of further Working Units
We have integrated additional working units into tmA2 and offer multiple facets of automation for the Sound Insulation Wall, Side Compactor, and DGS Roller Clamps.
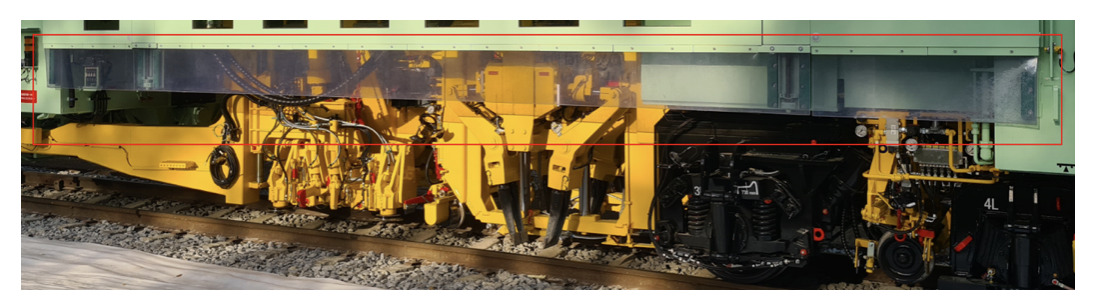
Doubling performance with 8×4 tamping units
So far the tmA2 has handled one sleeper at a time. Now we’ve doubled the performance – the algorithm behind the latest update for tmA2 can now handle two sleepers at a time. to support the 8×4 tamping multi-sleeper tamping units.
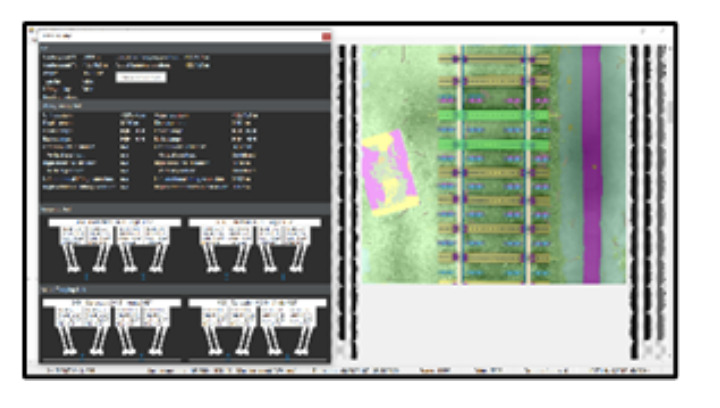
Tie Finders now supported in tmA2
We are integrating new sensors in the tmA2 to enhance its reliability. The tie finder is a sensor system to detect sleepers. In situations where sleepers are harder to detect, this special enhancement for track tamping machines comes in handy.
AI-controlled Lifting and Lining Unit
We used to rely on mathematical detection algorithms and have now completed the switch to a fully AI-controlled Lifting and Lining unit. This increases reliability and allows us to classify different objects within the web of rail, such as different types of joints or ATS-devices.
New Tamping KPI Reports
We have enhanced our tmA2 tamping protocols to now include KPIs. The KPIs currently focus on giving users a quick insight into how the system performed for them. It is now easy to see how many of the tmA2’s suggestions have been fully accepted without manual intervention of the operator. After all, the goal is to turn data into information and information into insight.
Introducing MLOps
Insights are also important during the development process, where tmc integrates MLOps practices to ensure the best for our customers. With automated validation of the models and automated build processes, delivery of new features can be done quickly with constant quality. As an important step, all decisions during the process are saved in a database, bringing light inside the Black Box AI and enabling manual influence.
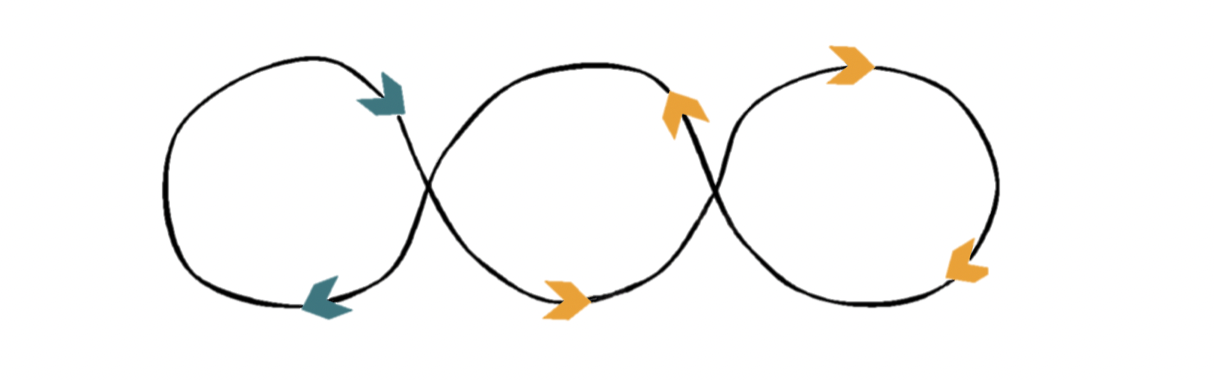
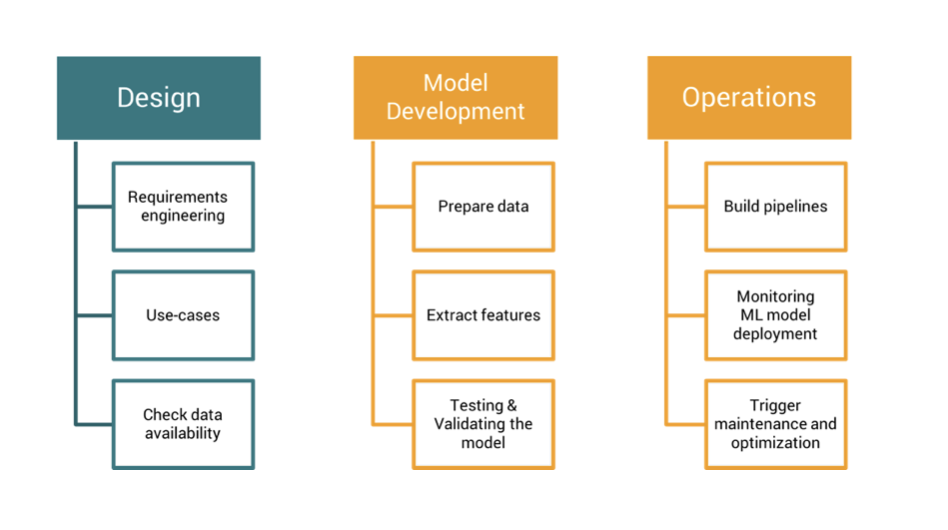